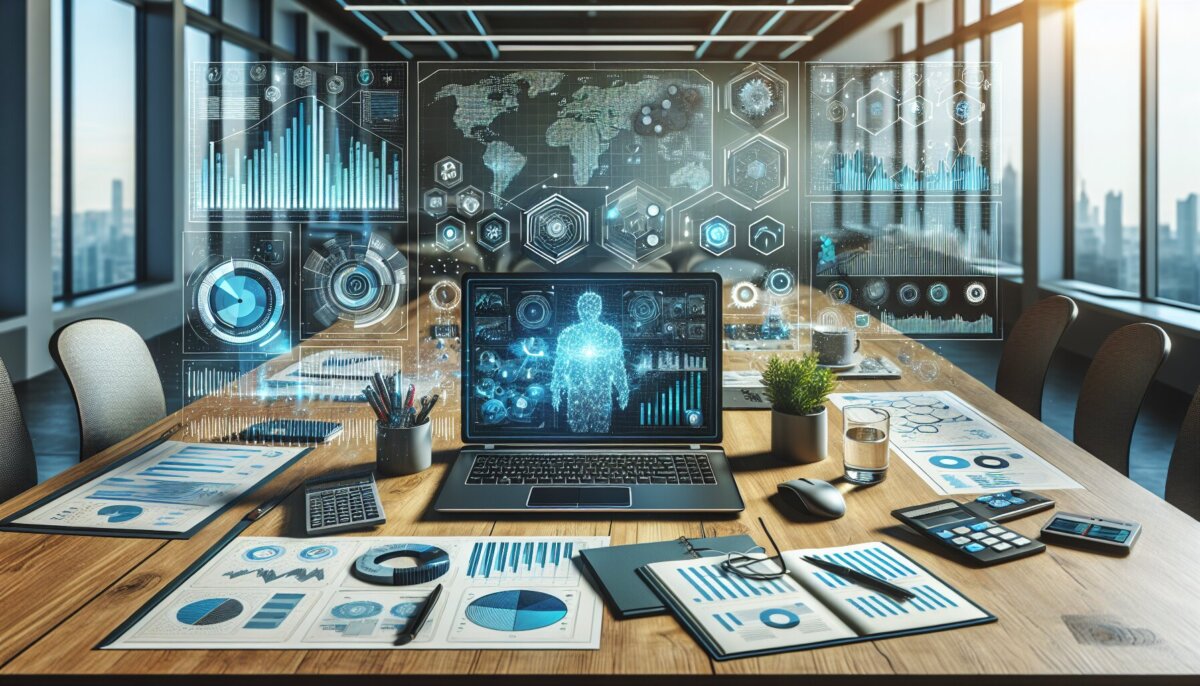
Author: Kysha Praciak
· 8 mins read · 414 views
Risk Reduction with Predictive Analytics
Predictive analytics, a sophisticated category of data analytics, leverages historical data, algorithm advancements, and machine learning techniques to forecast future outcomes, thus becoming a cornerstone in smart business practices[1][2]. With its foundation deeply rooted in statistical modeling, data mining, and employing algorithms like regression analysis, predictive analytics serves as a bridge between past data patterns and future possibilities, offering insights that can drive decision-making, enhance operational efficiency, and facilitate trend analysis[2].
This guide ventures into the realms of predictive modeling, illustrating its role in identifying business opportunities and mitigating risks. By harnessing the power of big data and predictive analytics, businesses can navigate the complexities of the marketplace with confidence. From banking to healthcare, and supply chain to marketing, this article will unveil how predictive analytics propels industries towards achieving heightened security, risk reduction, and overall smarter business practices.
Historical Data Analysis
Predictive analytics fundamentally relies on historical data to forecast future outcomes, employing mathematical models to discern key trends.
The methodology of predictive analytics is systematic, involving several critical steps:
- Selection of the Target Variable: Identifying the specific outcome to predict.
- Data Collection: Gathering relevant historical data.
- Data Splitting: Dividing the collected data into training sets for model building and test sets for validation.
- Model Selection and Input Configuration: Choosing the appropriate predictive model and configuring the input values.
- Model Validation: Testing the model to ensure accuracy.
- Implementation: Applying the model to make predictions.
Key Techniques in Predictive Analytics
- Predictive analytics incorporates various techniques such as regression, correlation, classification, segmentation, and clustering to analyze data and make predictions. Additionally, time series models are utilized to forecast future behavior based on historical data trends.
Integration of Machine Learning
- Machine learning enhances predictive analytics by providing advanced statistical methods for regression and classification, making the analysis more robust and insightful.
Streamlining Data Analysis
- Predictive analytics software streamlines the process by automating data collection from multiple sources, filtering data based on predefined criteria, and analyzing the data using diverse methodologies and algorithms.
Business Applications
- Predictive analytics serves as a crucial tool for business leaders, enabling them to forecast future trends and outcomes effectively. This capability is vital for strategic decision-making and gaining a competitive edge in the marketplace[3]. By employing techniques like demand forecasting and financial forecasting, businesses can predict future demands and financial performances, which are essential for planning and resource allocation[3].
Predictive modeling, a core aspect of predictive analytics, uses historical data to anticipate future trends and behaviors, aiding businesses in proactive planning. Techniques such as regression analysis, classification algorithms, and clustering methods play significant roles in predictive modeling by analyzing past data to predict future events and categorize new data[4].
Forecasting Future Trends
Predictive analytics is revolutionizing the way businesses forecast future trends, enabling them to anticipate market demands and customer behaviors with unprecedented precision. Utilizing advanced algorithms and big data, predictive analytics provides a robust foundation for making informed strategic decisions.
Here, we explore several key areas where predictive analytics is making a significant impact:
Demand Forecasting
- Passive and Active Forecasting: Businesses utilize both passive forecasting, which relies on historical data, and active forecasting, which considers potential future changes, to predict demand[3].
- Seasonal Trends: By analyzing past sales data, companies can prepare for seasonal fluctuations, ensuring optimal inventory management and marketing strategies[3].
- Macro Forecasting: External factors such as economic indicators and market trends are also analyzed to adjust forecasts and strategies accordingly[3].
Financial Forecasting
- Budgeting and Financial Planning: Predictive analytics aids organizations in forecasting future financial performance, which is crucial for budgeting and financial modeling[3].
- Risk Assessment and Management: By forecasting financial trends, companies can better manage risks, potentially saving substantial resources[3].
Customer Behavior Prediction
- Understanding Customer Needs: Predictive analytics helps businesses tailor their marketing strategies by predicting customer responses and preferences, thus enhancing customer satisfaction and loyalty[3].
- Risk and Opportunity Assessment: By analyzing customer data, companies can identify potential risks and opportunities, allowing for more targeted and effective business strategies[3].
Advanced Applications in Various Sectors
- Healthcare: In healthcare, predictive analytics optimizes resource allocation, improves patient care, and manages supplies more efficiently.
- Retail and E-commerce: Retailers use predictive analytics to optimize stock levels, predict consumer buying patterns, and enhance the customer shopping experience.
- Banking and Finance: Financial institutions leverage predictive analytics for better risk management, fraud detection, and customer service.
Predictive analytics continues to expand its influence across various industries, driven by technological advancements and the growing availability of data. As businesses increasingly rely on data-driven strategies, the role of predictive analytics in forecasting future trends becomes more critical, providing a competitive edge in the rapidly evolving market landscape.
Identifying Business Opportunities
Utilizing Predictive Analytics in Various Business Sectors
- Predictive analytics serves as a transformative tool across diverse sectors, enabling companies to forecast and adapt to market dynamics effectively. Financial institutions, industrial companies, logistic firms, insurers, retailers, and product development teams are leveraging predictive analytics for comprehensive risk management.
Broad Applications of Predictive Analytics
- Marketing Campaigns: Enhancing the effectiveness of marketing strategies.
- Operational Efficiency: Streamlining operations to boost productivity and reduce costs.
- Fraud Detection: Identifying and mitigating potential frauds before they occur.
- Customer Relationship Management (CRM): Improving interactions and relationships with customers.
- Recommendation Engines: Personalizing customer experiences by predicting preferences.
- Employee Retention: Predicting employee behaviors and improving retention strategies.
Industries Benefitting from Predictive Analytics
- Key industries such as aerospace, automotive, energy & utilities, banking and financial services, healthcare, retail, oil & gas, and manufacturing are harnessing predictive analytics to gain a competitive edge and drive innovation.
Enhancing Customer Understanding and Market Penetration
- Predictive analytics enables businesses to deeply understand customer behaviors and preferences, which assists in retaining existing customers and attracting new ones[3]. By analyzing extensive data sets, businesses can uncover hidden patterns and accurately predict consumer buying habits, thus identifying potential markets and new opportunities.
Market Analysis Through Data Analytics
- Data analytics tools are crucial for conducting thorough market analysis. These tools help businesses identify untapped opportunities by examining market trends, customer demographics, and competitor strategies. Additionally, analyzing customer data such as purchase history and online behavior provides insights into customer values, enhancing targeted marketing efforts.
Discovering Collaborative Opportunities
- Data analytics also facilitates the discovery of collaborative opportunities, enabling businesses to identify sectors or other businesses where partnerships could be mutually beneficial. This aspect of predictive analytics not only opens new avenues for growth but also helps in diversifying business risks.
Decision-Making and Efficiency Enhancement
- Business leaders utilize predictive analytics tools to integrate data-driven insights into their decision-making processes, thereby enhancing efficiency and minimizing risks[4]. This strategic application is evident across various functions within organizations, from retail marketing to human resources, where predictive analytics plays a pivotal role in forecasting customer preferences and anticipating operational needs[4][5].
Mitigating Risks with Predictive Analytics
Predictive analytics significantly enhances risk management capabilities within various business sectors. By integrating comprehensive data sets, both internal and external, businesses can proactively identify and mitigate potential risks, leading to more informed decision-making processes.
Key Benefits of Predictive Risk Insights Software
- Improved Decision-Making Capacity: Businesses can make more informed decisions by analyzing potential risks and their impacts.
- Lower Expenses: Effective risk management can lead to significant cost reductions.
- Enhanced Security and Effectiveness: Implementing predictive tools increases the overall security and efficiency of business operations.
Applications in Risk Management
- Predictive Maintenance: Optimizing maintenance schedules to reduce downtime and improve operational efficiency.
- Supply Chain Management: Addressing potential disruptions proactively to ensure smooth operations.
- Production Efficiency: Utilizing predictive analytics to enhance production processes and output.
Financial and Compliance Risk Management
- Predictive analytics tools enable businesses to manage financial risks by anticipating and mitigating potential financial disruptions and compliance issues, thus safeguarding against significant financial losses[10].
Fraud Detection in Banking
- Financial institutions leverage predictive analytics to develop robust fraud detection models. These models analyze vast amounts of data, including transaction histories and customer behavior patterns, to identify and prevent fraudulent activities effectively.
Ethical Considerations
- It is crucial to address ethical concerns such as data privacy, minimizing algorithmic bias, and maintaining transparency and accountability in predictive analytics applications, especially in sensitive sectors like banking and financial services.
Strategic Risk Management Framework
- Understand Your Risk Profile: Analyzing types and levels of risks to prioritize management efforts.
- Implement Risk Controls: Designing and implementing effective risk control systems and processes.
- Monitor and Measure Risk Performance: Continuously tracking the effectiveness of risk management strategies.
- Learn and Improve from Risk Events: Analyzing risk events to improve future risk management practices.
- Anticipate and Adapt to Risk Changes: Staying ahead of potential risk changes through continuous assessment and adaptation.
- Foster a Risk Culture: Developing a culture that supports proactive risk management across the organization.
Advanced Analytical Techniques
- Predictive and Prescriptive Analytics: Combining these techniques not only provides forecasts but also actionable insights for risk mitigation, enhancing the overall risk management framework.
- Scenario Evaluation: Empowering business leaders to assess various risk scenarios and formulate effective strategies using data-driven insights[3].
Practical Applications of Predictive Analytics
Predictive analytics has become a cornerstone in various industries by providing actionable insights through advanced models and integrated software.
Here are some practical applications across different sectors:
Healthcare
- Predictive analytics in healthcare not only aids in disease prediction but also enhances patient monitoring and care management. By analyzing symptoms and real-time data from IoT devices, healthcare providers can predict diseases and optimize patient treatment plans[5]. Furthermore, predictive maintenance of medical equipment ensures uninterrupted service provision[5].
Manufacturing
- In the manufacturing sector, predictive analytics plays a crucial role in preventing equipment malfunction. Algorithms analyze operational data to predict potential failures, allowing preemptive maintenance that saves significant costs and prevents production halts[2].
Entertainment & Hospitality
- The entertainment and hospitality industries benefit from predictive analytics by optimizing staffing needs. For example, Caesars Entertainment uses predictive models to forecast staffing requirements, ensuring efficient operation and customer satisfaction[2].
Marketing
- Predictive analytics transforms marketing strategies by predicting sales trends and customer behaviors. This enables businesses to tailor their marketing campaigns more effectively and target ads based on the predicted likelihood of customer progression through the sales funnel[2][4].
Internet of Things (IoT)
- IoT applications in predictive analytics include monitoring equipment to predict failures and optimizing energy use by forecasting peak demand times. This not only enhances operational efficiency but also promotes sustainability[5].
Social Media Analytics
- By analyzing data from social media platforms, predictive analytics can gauge customer sentiments and market trends. This information is crucial for businesses to adjust their strategies in real-time, ensuring they meet the current market demands[5].
Each of these applications demonstrates the versatility and impact of predictive analytics across different fields, highlighting its role in driving operational efficiency, reducing risks, and enhancing profitability.
Conclusion
Throughout this exploration, we’ve seen how predictive analytics stands as a transformative force across various sectors, offering businesses the ability to foresee and adapt to future challenges and opportunities with remarkable precision. By drilling down into historical data, employing sophisticated algorithms, and leveraging machine learning techniques, companies can not only enhance operational efficiency but also identify and mitigate risks proactively. This strategic foresight allows for smarter business practices, enabling organizations to maintain a competitive edge in the ever-evolving marketplace.
The implications of employing predictive analytics extend far beyond simple risk reduction, touching on aspects such as customer satisfaction, product innovation, and strategic planning. As industries continue to navigate through complex data landscapes, the role of predictive analytics in shaping future trends cannot be understated. It empowers businesses to make informed, data-driven decisions that foster growth and sustainability in the long term. Thus, as we look forward, the integration of predictive analytics into business strategies is not just advisable; it’s indispensable for those aiming to thrive in tomorrow’s market dynamics.
Looking for Expert IT Solutions?
Subscribe to Our Newsletter for Exclusive Tips and Updates!
Stay ahead of tech challenges with expert insights delivered straight to your inbox. From solving network issues to enhancing cybersecurity and streamlining software integration, our newsletter offers practical advice and the latest IT trends. Sign up today and let us help you make technology work seamlessly for your business!
FAQs
What does predictive analytics entail within the realm of risk management?
Predictive analytics involves analyzing both structured and unstructured data to forecast market trends and economic behaviors. This advanced approach is transforming how risk management is conducted in the finance sector, enabling investors and fund managers to make more informed decisions.
How does predictive analytics contribute to business intelligence?
Predictive analytics plays a crucial role in business intelligence by enhancing fraud detection. It allows companies to identify and thwart fraudulent activities, thereby protecting against financial losses. For instance, credit card companies use machine learning algorithms that monitor purchasing patterns to flag any unusual transactions that may suggest fraud.
Are there any drawbacks associated with predictive analytics?
One significant downside of predictive analytics is that despite having ample data, critics point out that algorithms may not fully account for various unpredictable factors such as weather changes, human emotions, or interpersonal dynamics, which can all impact consumer behavior.
What is the fundamental objective of predictive analytics?
The primary goal of predictive analytics is to utilize data, statistical algorithms, and machine learning to predict future events based on past data. It aims to extend the understanding of past occurrences to make educated estimations about future events.
References
[1] –https://www.cio.com/article/228901/what-is-predictive-analytics-transforming-data-into-future-insights.html
[2] –https://online.hbs.edu/blog/post/predictive-analytics
[3] –https://online.mason.wm.edu/blog/predictive-analytics-in-business
[4] –https://onlinedegrees.scu.edu/media/blog/applications-of-predictive-analytics-in-business
[5] –https://pwskills.com/blog/predictive-analytics-examples/
Share