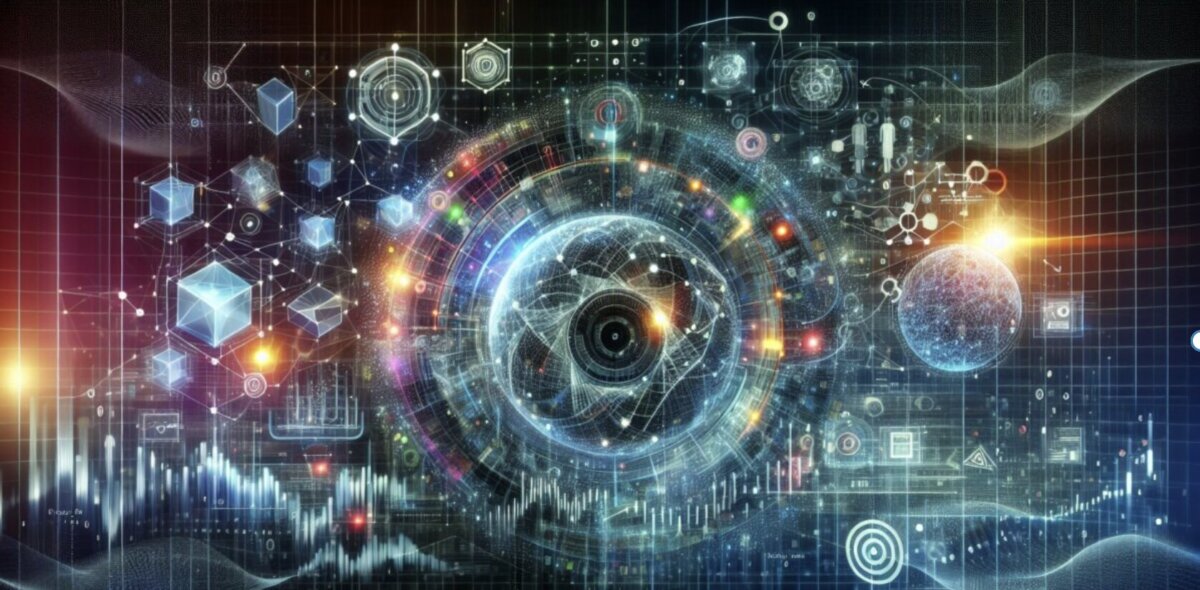
Understanding the Dynamics of Pattern Recognition in Predictive Analytics
Author: Kysha Praciak
· 5 mins readPattern recognition, a cornerstone of artificial intelligence, leverages machine learning to identify and predict hidden or subtle patterns in data[1][2]. It equips your systems to classify unseen data, make valuable predictions, and apply learning techniques effectively. Essential across various applications, from facial recognition to tumor detection, pattern recognition strengthens predictive analysis by bridging the gap between incoming data and stored knowledge.
By understanding pattern recognition’s role, you enrich your knowledge in predictive modeling and predictive analysis, providing a robust framework for data analysis. This article dives into the dynamics of pattern recognition, exploring its techniques, practical applications, challenges, and future trends in predictive analytics. With pattern recognition’s capacity to process information at multiple angles and distances, you’re poised to grasp how this technology advances the field of predictive modeling.
The Role of Repetitive Analysis in Predictive Modeling
Repetitive analysis plays a pivotal role in enhancing the accuracy and reliability of predictive modeling. This process involves several key steps and methodologies that ensure the predictive models remain current and effective:
- Structured Framework for Repetitive Analysis:
- Observation Window: Utilizes a dynamic approach to select the time frame for data analysis, crucial for capturing relevant trends and changes in the data set.
- Raw Data Processing: Involves the extraction of features directly from the data, ensuring that the information fed into the model is accurate and pertinent.
- Modeling Type: Selection between various modeling approaches, including statistical algorithms and machine learning techniques, to best suit the data and prediction objectives.
- Methodological Frameworks:
- Several frameworks have been identified for integrating repeated measurements in predictive models, such as time-dependent covariate modeling, generalized estimating equations, and machine learning, each offering unique advantages in handling longitudinal data.
- Importance of Regular Monitoring:
- To prevent model degradation, regular evaluation is essential. The 20/20/20 guideline suggests model rebuilding when significant changes in the data or the population being modeled are observed, ensuring the predictive model remains relevant and accurate.
These steps and methodologies underscore the importance of repetitive analysis in maintaining the efficacy of predictive models, making it a cornerstone of predictive analytics.
Techniques for Repetitive Analysis in Pattern Recognition
In the exploration of techniques for repetitive analysis in pattern recognition, several methodologies stand out due to their efficacy and widespread application across different fields:
- Classification and Clustering: At the core, pattern recognition involves the classification and clustering of patterns, where classification sorts data into predefined categories, and clustering groups data points based on their similarities. This duality is fundamental in understanding and predicting behaviors and outcomes.
- Advanced Techniques:
- Neural Networks: Mimicking the human brain’s structure, neural networks excel in complex tasks like image and speech recognition. Their ability to learn and adapt makes them invaluable for predictive modeling and pattern recognition.
- Decision Trees: These are used for both classification and prediction, offering clear, logical structures for decision-making processes. Their application ranges from financial predictions to image recognition, showcasing versatility.
- Statistical Pattern Recognition: This technique relies on statistical analysis to identify patterns within data, especially useful in image analysis where human observation might not suffice.
- Problem-Solving Steps: Addressing pattern recognition problems involves a systematic approach: data collection, understanding the problem and its classes, and classifying, measuring, and annotating the data. This structured method ensures a comprehensive analysis and application of insights[3].
These techniques, coupled with a methodical approach, form the backbone of repetitive analysis in pattern recognition, offering a blend of traditional and advanced methods to tackle complex predictive modeling challenges.
Practical Applications of Repetitive Analysis
Pattern recognition’s practical applications span across various industries, significantly enhancing operational efficiency and decision-making processes:
- Healthcare:
- Predictive modeling uses machine learning to analyze historical health data, predicting future health events and improving patient outcomes. This approach is instrumental in detecting diseases early, optimizing healthcare operations, and enhancing key performance indicators (KPIs).
- In critical care settings, incorporating repeated measurements into multivariable prediction research helps in making more accurate prognoses and treatment decisions.
- Finance and Insurance:
- Analyzing demographics, past payment histories, and customer support logs enables predictive analytics to assess creditworthiness or predict fraudulent activities. This capability is crucial for managing risks and preventing fraud in both sectors.
- Manufacturing:
- Predictive analytics monitors equipment conditions and predicts failures before they occur, preventing costly downtimes and maintaining continuous production flow. This application is a key component of predictive maintenance strategies.
These examples underscore the versatility and impact of pattern recognition and predictive analytics across different domains, driving advancements and innovations that cater to specific industry needs and challenges.
Need Expert IT Solutions?
Get a Free Consultation Today!
Whether you’re dealing with network issues, cybersecurity concerns, or software integration, our team of IT experts is here to help. Don’t let tech troubles slow you down. Call us now for a complimentary initial assessment, or click below to fill out our quick contact form. Let’s make technology work for you.
Challenges and Solutions in Repetitive Analysis
In addressing the challenges inherent in repetitive analysis for pattern recognition, it’s crucial to understand and mitigate the obstacles to ensure the accuracy and reliability of predictive modeling. The challenges and their corresponding solutions can be categorized as follows:
- Data Complexity and Volume:
- Challenge: Identifying and extracting key points from complex data sets pose significant difficulties, with risks of overlooking crucial information or misinterpreting data due to its volume or complexity.
- Solution: Implementing advanced machine learning algorithms and utilizing neural network analysis can help in discerning patterns and changes not immediately apparent, thereby managing the vast and complex datasets more effectively[4].
- Accuracy and Reliability:
- Challenge: Ensuring the precision of extracted factual information, such as pricing details and quantitative metrics, is essential but challenging, especially when data is dense or imbalanced.
- Solution: Regularizing techniques, robustness measures, and the integration of statistical methods with machine learning can enhance the model’s reliability and accuracy, addressing issues like overfitting and the curse of dimensionality.
- Comprehensiveness and Clarity:
- Challenge: Distilling complex information into a clear, concise summary while considering both directly and indirectly related aspects is challenging but necessary for comprehensive analysis.
- Solution: Visualization techniques aid in presenting summaries in a clear, distinct, and grouped format, significantly improving understanding and decision-making. Additionally, considering the relevance of information broadly ensures a more comprehensive analysis[2].
By tackling these challenges with targeted solutions, repetitive analysis in pattern recognition can be optimized, enhancing the predictive modeling process and its outcomes.
Future Trends in Repetitive Analysis and Predictive Modeling
Exploring the future trends in repetitive analysis and predictive modeling reveals a landscape where technological advancements and innovative methodologies are poised to redefine the field:
- Integration of Advanced Technologies:
- Artificial Intelligence (AI) and Machine Learning (ML): The integration of AI and ML with predictive analytics is set to become more sophisticated, enabling the analysis of complex datasets with higher accuracy and efficiency.
- Big Data Analytics: Leveraging big data will enhance the capability of predictive models to process and analyze vast amounts of data, leading to more accurate predictions.
- Predictive Analytics Software Evolution:
- 2024 Leading Tools: Tools such as SAP Analytics Cloud, Alteryx AI Platform, and IBM Watson Studio are expected to evolve, offering more powerful and user-friendly analytics solutions. These tools will likely incorporate more advanced AI capabilities and better data integration features[4].
- Software Selection: The choice of predictive analytics software will increasingly depend on specific industry needs, with a focus on those offering comprehensive data connectors, robust AI/ML capabilities, and competitive pricing[4].
- Focus on Healthcare and Education:
- Healthcare: Predictive modeling in healthcare will continue to grow, especially in utilizing Electronic Health Records (EHR) for patient data analysis. This will aid in early disease detection, personalized treatment plans, and overall healthcare system efficiency.
- Education: Predictive learning analytics will play a crucial role in improving learning outcomes in higher education. By analyzing student performance, engagement, and other metrics, educational institutions can tailor their teaching methods and interventions to better meet student needs.
These trends underscore the dynamic nature of repetitive analysis and predictive modeling, highlighting the importance of staying abreast of technological advancements and industry-specific applications to harness the full potential of these powerful analytical tools[4].
Conclusion
Through this exploration, it has become evident that pattern recognition within predictive analytics heralds a profound impact across various domains, including healthcare, finance, manufacturing, and more. The blend of methodologies and technologies, from neural networks to decision trees and beyond, underpins the dynamic capability of predictive analytics to analyze, interpret, and predict data patterns with remarkable accuracy. This capability not only enhances operational efficiency and decision-making processes but also paves the way for innovations tailored to the specific needs and challenges of different industries.
Looking ahead, the continual integration of advanced technologies like artificial intelligence, machine learning, and big data analytics spells a promising future for repetitive analysis and predictive modeling. The evolution of predictive analytics software and the focus on sectors such as healthcare and education are indicative of the broadening scope and deepening impact of these analytical methods. As technology advances, staying informed and adaptable will be key for leveraging the full potential of predictive analytics, ensuring its benefits are maximized across the board.
References
[1] –https://www.linkedin.com/pulse/repetition-solution-guy-reams
[2] –https://www.linkedin.com/pulse/pattern-recognition-predictive-analytics-russ-penlington
[3] –https://www.linkedin.com/advice/0/how-can-pattern-recognition-used-solve
[4] –https://www.linkedin.com/pulse/power-predictive-analytics-leveraging-data-future-success
Share